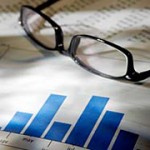
In the business sector, companies have been using predictive analysis for years to improve performance, predict stocks, or take action and change direction when troubling trends appear. They gather data from a variety of sources and use modeling to pinpoint disturbing developments, identify where things might be headed, and make appropriate changes.
The public sector typically lags behind business: While it has become relatively common within education to use data analysis for tracking and measuring performance at the school, educator, and student levels, far fewer schools and colleges have taken analytics to the next level–using advanced analytics strategies to identify trends that can help predict future performance and help school leaders make key decisions, early on in the process, that can change a potentially unwelcome outcome or take advantage of a positive trend.
But that’s beginning to change. Using advanced analytics software, the University of California system has saved $167 million in the last five years by mitigating risks across its 10 campuses and five medical centers, for example–and the Houston Independent School District has saved millions of dollars in labor and expenses for food service, transportation, and other critical functions (see “HISD sees 151-percent ROI in 10 years from analytics project” and “UC campuses mitigate risk–and save millions in the process.”)
These are just a few of the ways that schools are tapping into this trend to improve their operations.
Why advanced analytics?
Some school leaders are beginning to realize what can be done with advanced analytics and have started taking data analysis to a deeper level in order to mine trends to their fullest extent.
“As a society, we’ve always been fascinated with the idea of predicting. The movie Minority Report, for example, was about predicting where crime was going to occur,” says Stephen Gold, an executive in the global education business unit for SPSS products at IBM. “As a parent, predicting makes so much sense: Don’t tell me my kid failed a class after it’s already happened.” It’s far better, he says, if a parent can be warned in advance if his or her child is at risk of failing a subject or a grade.
IBM offers tools, such as SPSS Modeler, that can help schools and colleges improve outcomes–both on the education side and the business side–by identifying trends and allowing administrators to make decisions based on patterns and associations found within their data.
For example, says Gold, someone using SPSS Modeler could look at attendance, tardiness, and visits to the school nurse, in conjunction with teacher attendance, to see how often a student is actually in class at the same time as the teacher, and to see how all these factors work together to affect student performance. (See “Helping schools forecast future trends.”)
School leaders could use the software to answer an endless number of questions.
For example: What’s the most important characteristic of a good instructor–advanced degrees, national certification, or some other characteristic? If a student is habitually in the nurse’s office at a particular time of day, what bearing will that have on the success of the student? Which instructors are the best at teaching certain types of students? What is the best learning environment for students with special needs? What’s the best course of action to take for a student who scored poorly on a biology test at the beginning of the year, to ensure that he passes at the end?
Administrators and educators then can make decisions that can change a potentially negative outcome before it takes place. (See “Tennessee district improves student performance, reduces dropout rates.”)
Using advanced analytics for predictive modeling marks a total structural change in education. “It gives us the ability to act,” Gold says.
Using ‘imperfect data’
While many schools have become adept at tracking and measuring student and teacher performance, structured information such as grades, state test scores, and attendance–as well as other information, such as food service data and bus routing schedules–is typically kept in a variety of different repositories.
Then there’s the high volume of unstructured data that can exist within a school or district: information about disciplinary action, parent-teacher reviews, notes from a counselor, surveys of parents or students, or notes from PTA members about different issues affecting a school, to name a few.
Data mining tools such as SPSS Modeler or SAP’s BusinessObjects analytics allow institutions to pull together both structured and unstructured data. And that’s important, because 80 percent of the data produced every day–from eMail messages to call logs to blogs on the internet–are unstructured, according to Gold.
“Generally, the information is there. There just hasn’t been a vehicle by which institutions could take advantage of it,” he says. “Now, text analytics products are looking at sentences, how the words appear, what the words mean.”
Then, they build relationships between those words and make connections, such as identifying students who indicate they’re always tired, or students who say they have little support, and predict how those factors affect test scores.
Without the appropriate tools, school organizations are unable to use methods such as these, and qualitative factors end up being analyzed in a subjective fashion, with nobody pulling together insights based on all the data–which leads to wasted opportunities.
But as the use of advanced analytics becomes more widespread, the trend is moving from simple reporting to predicting. “It changes the school’s mindset from thinking about, ‘What do I do with at-risk kids?’ to ‘How do I keep kids on track so they don’t become at-risk?’” says Gold. “If I provide early intervention, I can preempt or prevent completely what will be the likely outcome.”
Another benefit of a product like SPSS Modeler is that it provides real-time data, and it puts the data into the hands of those making the decisions. Administrators and educators no longer have to ask the IT department for data and wait weeks for this information to be returned to them, by which time it is old and perhaps no longer useful.
Gone, too, is the need to dump data from a variety of different systems into a giant data warehouse every night–an important point, as data in that case are “only as accurate as the last time you did the dump,” points out Don Seaman, business development director at SAP Public Services, another provider of predictive modeling solutions.
Rather than dumping information into a data warehouse on a regular basis, educators and administrators can immediately begin using the information to make business and education decisions.
“That’s the power [of predictive modeling], going from hindsight to insight to foresight,” says Sherry Amos, executive director for industry strategy at SAP Public Services. “You can access the data where [they exist]. You don’t have to spend months pulling [the data] together.”
With data mining of this sort, there’s also no need to form a supposition about what you think the data will show. Rather, educators can wait to see what trends the mining process uncovers.
“With statistics, you have to have really good data and a hypothesis,” explains Gold. “You have to say, ‘I believe this type of student, with this type of history, will exhibit this type of behavior.’”
And that’s difficult, he says, because data often are in silos. Also, not all schools have that kind of continuity with a student, as students often transfer from one school to another.
A good advanced analytics software program “allows you to take whatever data you have and allows the technology to work through the information and see what the outcome is. You don’t need a hypothesis,” Gold says.
What you need to get started
If perfect data and a fully formed hypothesis are not needed to begin using predictive modeling, what is needed?
“You need to understand the metrics you’ll want to be gathering and the outcomes you’re looking to achieve, and [you need to know] the data required to track [these] so you can show measurable progress,” Seaman says.
That, he says, requires a highly collaborative effort, with participants “from the central office all the way out to the individual schools” or departments.
Schools that have invested in building out a more robust data offering, and that have begun aggregating student components of data records, test scores, and other information, will benefit from having all this information pulled together. But even schools that haven’t had the resources to do that can begin to use predictive analytics.
“There’s no prescriptive that says you have to have a certain amount of data before you’ll be able to get value,” says Gold.
“You can have five years of longitudinal data in one area, two years of data here, eight years there, and that’s great. Just start,” he suggests.
Another important element in getting started with predictive analysis is a group of people with advanced analytics training. “Finding individuals with these skills is a prerequisite for doing these things at an institutional level,” Gold says.
In terms of getting buy-in from the top, it can help to start small, says Amos. Rather than say you’re going to predict student achievement across the board and come up with a prescription for improving success, pick a specific area on which to focus.
You might measure on-time arrival of buses, for example: Choose key performance indicators to measure bus arrival time; determine what’s good, fair, or poor in terms of bus arrivals; and begin to analyze whether and how that has an effect on student performance. Then, you can begin making decisions on how to improve those numbers–after which you can move on to another area of focus.
“These are relatively short processes,” Amos says. “They’re not multiyear projects. It does a lot to show small successes early to bring the cultural environment along. And these are projects that can be funded in small increments.”
Another good place to start is driving cost savings.
Using advanced analytics often starts on the business side, looking at strategic areas of an institution’s mission to see where improvements can be made. “Cost savings are the low-hanging fruit,” says Amos–and top-level decision makers are more likely to budget for a new software program if it can show immediate financial benefits.
Ways to use predictive modeling
The uses of predictive modeling can be endlessly varied, and they can run the gamut from improving efficiencies to saving money to enhancing student achievement.
Just a few examples include:
• Late buses, the early breakfast program, and their impact on learning success: If buses arrive late in the mornings, students might not be able to participate in the breakfast meal program–and students are not ready to learn if they don’t eat in the mornings. Typically, the breakfast meal program and the bus program are tracked by two different groups and in two different databases. But with predictive analytics, administrators can see how they work together and whether those two factors have an impact on learning.
• Identifying problems: One school district noticed that a single group of students was continually late to school every Wednesday morning. By applying IBM’s advanced analytics, administrators discovered that the parents of this group of students all worked the late shift on Tuesday night, then the early shift on Wednesday morning. The kids were staying up late on Tuesdays, then falling back asleep on Wednesday mornings after their parents woke them up and left for work, says Rob Dolan, a worldwide industry executive in business analytics for IBM. After identifying the problem, administrators were able to contact the parents and explain the situation, which was then rectified.
• Course planning: An instructor’s plan can be shaped, day to day, by data points. Imagine that an instructor has a lesson plan for Tuesday, teaches the class, then inputs feedback from Tuesday’s exercise into the system. That teacher might find that four of the five concepts taught on Tuesday resonated well with the students, but the fifth concept was a problem. That could suggest that the lesson plan for Wednesday focus on that fifth concept. “It becomes part of a process, changing activities in a classroom to provide for a better experience,” Gold says.
• College recruitment and retention: As many colleges and universities struggle with retention problems–students who finish their freshman or sophomore year and then drop out–some have used advanced analytics to discover what types of students they should be recruiting to find the attributes that make a student most likely to complete his or her college career. Institutions of higher learning also can look at what types of programs are most likely to help keep a student engaged. They can identify, for example, that “this type of student who lives in this dorm and with this major will most likely stay [in school] if I offer them this club,” explains David P. Whirlow, a senior managing consultant in business analytics and optimization for IBM.
• Optimizing the scheduling of classrooms: Syracuse University has used IBM’s advanced analytics to help schedule its facilities for classes, taking into account how students are moving between classrooms and the best way to schedule within the university’s budget constraints.
• Optimizing alumni donations: Another higher-education application of advanced analytics would be using the method to discover the best way to interact with the alumni community to boost donations.
Reaching the nirvana of ‘personalized instruction’
In recent years, the pie-in-the-sky ideal of learning has been the notion of individualized lesson plans. Through advanced analytics, the education industry is getting closer to that ideal.
Periodic assessments of a student’s knowledge, combined with other data such as the challenges specific to that student, the student’s demographic information, and information about his or her instructor (what type of certification the teacher has, how often he or she is absent, subject matter background, etc.), can help school leaders understand not only how a student is performing but how a student will perform under certain circumstances. This creates the opportunity to formulate specific lesson plans for that student.
“Picture Johnny with a Kindle, and what Johnny does on Wednesday is different from what Mary does on Wednesday,” Gold explains. “Their actions are shaping their own different lesson plans as we create a personalized experience.”
Jennifer Nastu is a freelance writer living in Colorado, who writes frequently about education and technology.
- Research: Social media has negative impact on academic performance - April 2, 2020
- Number 1: Social media has negative impact on academic performance - December 31, 2014
- 6 reasons campus networks must change - September 30, 2014
Comments are closed.